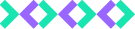
Videos
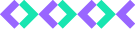
Data-driven services in the Data Space
Want to learn more about the Enershare services designed by project partners? View the demos here.
Demand Response (Injected Frequency)
Project Partner: Fortum
Demonstration of the Demand Response service controlling a fleet of simulated EVSEs that are provided by the EV Aggregator service. The frequency signal is simulated so that it results in repeated full FCR-D Up activations and deactivations, and we can see that the EVSEs are responding to commands issued by the Demand Response service in real-time. The services are connected to each other using the TSG connector under the Enershare Dataspace.


Demand Response (Real Frequency)
Project Partner: Fortum
Demonstration of the Demand Response service controlling a fleet of simulated EVSEs that are provided by the EV Aggregator service. Additionally, the frequency signal used as input is provided by the Grid Frequency service, and it reflects the live frequency of the power grid in Sweden at the time of recording. Unfortunately, the frequency never entered the activation zone for FCR-D Up during the recording, so no activation/deactivation commands were observed and the simulated fleet kept its consumption constant. The services are connected to each other using the TSG connector under the Enershare Dataspace.


Electric Mobility Platform
Project Partner: Emotion
EMP enables a cooperation mechanism between DSOs, CPOs and EV users. DSO monitors the electricity grid and, thanks to accurate forecasting systems, is able to identify how, when and where to charge electric vehicles to avoid congestion problems. CPO will thus be able to provide a dynamic charging price based on DSO needs, to offer an advantageous charging price in charging stations located in congested areas and attract a greater number of EV users increasing its revenue.


Health Insurance Alarms for Seniors Living Alone
Project Partner: Smart Energy Lab
Monitor energy consumption in households with seniors living alone, identify regular usage patterns, and trigger alarms when there are significant changes from these patterns.


Energy Usage Prediction Service
Project Partner: ComSensus
A service that generates typical historical daily profiles for electrical and district heating consumption for various household types. It also offers next-day forecasts based on location data and weather forecast.


Multivariate Energy Time Series Forecasting
Project Partner: INESC TEC
Data-space integrated Vector Autoregressive model for short-term forecasts of renewable energy and load using real-time data from geographically distributed sensors, wind turbines, and solar power inverters, considering vertical federated learning.


Anomaly Detection of Electric Generator
Project Partner: TECNALIA and HINE
Service for detecting electric anomalies in the stator winding of synchronous generators with permanent magnet technology, specifically designed for operation in wind turbines. It has been developed and validated with generators of 850kW of rated power.


Anomaly Detection of Hydraulic Pitch System
Project Partner: TECNALIA and HINE
Service for detecting anomalies in hydraulic pitch system: piston cylinder seal leak, excessive friction in cylinder, low pre-charge in service accumulator, low flow in pump.


Digital Twin of Electric Generator
Project Partner: TECNALIA and HINE
This digital twin allows the generation of synthetic data of synchronous generators with permanent magnet technology, specifically designed for operation in wind turbines. It has been developed and validated with generators with 850kW of rated power.


Digital Twin of Hydraulic Pitch System
Project Partner: TECNALIA and HINE
This digital twin allows the generation of synthetic data of hydraulic pitch systems. It has been developed and validated with the DTU 10MW Reference Wind Turbine.


Architecture and Edge Integration of Pilot 1 Services in the DS
Project Partner: TECNALIA and HINE
It shows the general integration architecture of Pilot 1 services in the data space, and the specific edge integration.


Flexibility Analytics Register (FAR) Service
Project Partner: European Dynamics
The Flexibility Analytics and Register Service (FAR) intends to provide a two-fold service that aims to support Flexibility Service Providers (i.e., Aggregators, end-users, but namely actors’ with a certain knowledge of managing an energy portfolio) for i) managing their portfolio in a streamlined manner (uploading fresh time-series data), registering a assets and managing them on groups, all via the graphical user interface, ii) obtain user-specific analytics via requesting the FAR service to post-process the data owned by the user as well as analytics related to grids (i.e., grid needs) and flexibility analytics by considering aggregated FSP data.


Cross-operators’ Portal (COP) Service
Project Partner: European Dynamics
The COP intends to provide a two-fold service that aims to support grid operators (i.e., in this case Pilot case for District Heating Operator (DHO) and the DSO) for i) obtaining comprehensive analytics on the installed flexibility and the spatio-temporal flexibility availability across their grids, ii) allow them the to announce/post flexibility needs (defined as quantity; product-service type) which is communicated via the OneNet connector to the FAR users, but also to populate (amongst each other DSO-DHO) cross-energy flexibility sharing.


Federated Learning Approach to an Anomaly Detection Service
Project Partner: Fraunhofer
Uses autoencoders to learn the normal behaviour of wind turbines using historical SCADA data. After the training of the autoencoders, a threshold is fitted to the reconstruction error of the normal behaviour data. By comparing this threshold to the reconstruction errors of new input data, the anomaly detection framework can differentiate between normal behaviour and anomalous patterns.


Substation Load Forecasting Tool
Project Partner: R&D Nester
The service provides day-ahead net load forecasts. It can be applied to nation-wide aggregate net load prediction, to all the way down to domestic level net load forecasting.. It does this by leveraging on a Stacking Ensemble of ML models, which, using past net-load data and features extracted from said data, is able to negate the biases of each of the stack members and make an improved forecast than any of the member models.


Federated Transfer Learning Flexibility Potential Service
Project Partner: ComSensus
This service primarily orchestrates the life cycle of flexibility potential AI models. In the scenario, we create a digital twin of energy substations, each with its own characteristics and local data. To deploy the AI model to these substations to start assesing the flexibility, we first download the general flexibility model from the general storage and we retrain the general model to the local data through the process of transfer learning. Where afterwards they are orchestrated with our system to ensure the correct life cycle of the AI models deployed for flexibility assesment and helps the operators keep the grid free of problems.

